
搜索网站、位置和人员

新闻与活动 活动信息
西湖名师论坛第七十期 | Yew-Soon Ong: Generalize Optimization Intelligence
时间
2019年12月16日(周一)
上午10:00-11:30
地点
西湖大学云栖校区5号楼一楼学术报告厅
主持
西湖大学工学院人工智能与机器人中心研究员 于长斌
受众
全体师生
分类
学术与研究
西湖名师论坛第七十期 | Yew-Soon Ong: Generalize Optimization Intelligence
时间:2019年12月16日(周一)上午10:00-11:30
Time:10:00-11:30 AM, Monday, Dec 16, 2019
地点:西湖大学云栖校区5号楼一楼学术报告厅
Venue:Lecture Hall, 1F, Building 5, Yunqi Campus
主持人:西湖大学工学院人工智能与机器人中心研究员 于长斌
Host:Changbin Yu, Ph.D., PI of School of Engineering, Westlake University
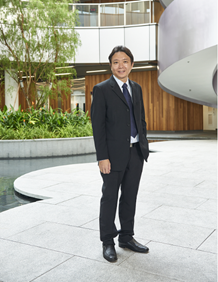
主讲人/Speaker:Yew-Soon Ong, President’s Chair Professor, School of Computer Science and Engineering, Nanyang Technological University (NTU); Chief Artificial Intelligence Scientist of the Agency for Science, Technology and Research Singapore; Fellow of IEEE
Prof. Ong received the Ph.D. degree in artificial intelligence in complex design from the University of Southampton, U.K., in 2003. At NTU, he serves as Director of the Singtel-NTU Cognitive & Artificial Intelligence Joint Lab. His research interests in artificial and computational intelligence. He is founding Editor-in-Chief of the IEEE Transactions on Emerging Topics in Computational Intelligence and AE of IEEE Transactions on Neural Networks & Learning Systems, the IEEE Transactions on Cybernetics, IEEE Transactions on Evolutionary Computation and others. He has received several IEEE outstanding paper awards and listed as a Thomson Reuters highly cited researcher and among the World's Most Influential Scientific Minds.
报告题目/Title:
Generalize Optimization Intelligence
讲座摘要/Abstract:
Traditional Optimization tends to start the search from scratch by assuming zero prior knowledge about the task at hand. Generally speaking, the capabilities of optimization solvers do not automatically grow with experience. In contrast however, humans routinely make use of a pool of knowledge drawn from past experiences whenever faced with a new task. This is often an effective approach in practice as real-world problems seldom exist in isolation. Similarly, practically useful artificial systems are expected to face a large number of problems in their lifetime, many of which will either be repetitive or share domain-specific similarities. This view naturally motivates advanced optimizers that can replicate human cognitive capabilities, leveraging on lessons learned from the past to accelerate the search towards optimal solutions of never before seen tasks. With the above in mind, this talk aims to shed light on recent research advances in the field of global black-box optimization that champion the general theme of ‘Generalizable Optimization Intelligence’. A brief overview of associated algorithmic developments in memetic computation and Bayesian optimization shall be considered, with illustrative examples of adaptive knowledge transfer across problems from diverse areas, including, operations research, engineering design, and neuro-evolution.
讲座联系人/Contact:
科技合作部 毕老师
biguanying@westlake.edu.cn